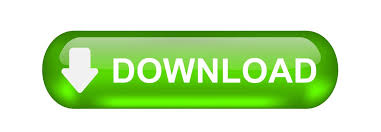

randn ( 1, 1, 3 )) # clean out hidden state out, hidden = lstm ( inputs, hidden ) print ( out ) print ( hidden )Įxample: An LSTM for Part-of-Speech Tagging ¶ view ( len ( inputs ), 1, - 1 ) hidden = ( torch. the second is just the most recent hidden state # (compare the last slice of "out" with "hidden" below, they are the same) # The reason for this is that: # "out" will give you access to all hidden states in the sequence # "hidden" will allow you to continue the sequence and backpropagate, # by passing it as an argument to the lstm at a later time # Add the extra 2nd dimension inputs = torch. # the first value returned by LSTM is all of the hidden states throughout # the sequence. view ( 1, 1, - 1 ), hidden ) # alternatively, we can do the entire sequence all at once. # after each step, hidden contains the hidden state. randn ( 1, 1, 3 )) for i in inputs : # Step through the sequence one element at a time. LSTM ( 3, 3 ) # Input dim is 3, output dim is 3 inputs = # make a sequence of length 5 # initialize the hidden state. Part-of-speech tags, and a myriad of other things. We can use the hidden state to predict words in a language model, There is a corresponding hidden state \(h_t\), which in principleĬan contain information from arbitrary points earlier in the sequence. In the case of an LSTM, for each element in the sequence, So that information can propagate along as the network passes over the For example, its output could be used as part of the next input, Another example is the conditionalĪ recurrent neural network is a network that maintains some kind of The classical example of a sequence model is the Hidden Markov Models where there is some sort of dependence through time between your Sequence models are central to NLP: they are There is no state maintained by the network at all. Sequence Models and Long Short-Term Memory Networks ¶Īt this point, we have seen various feed-forward networks.
#Finetune automatic music tagger android#

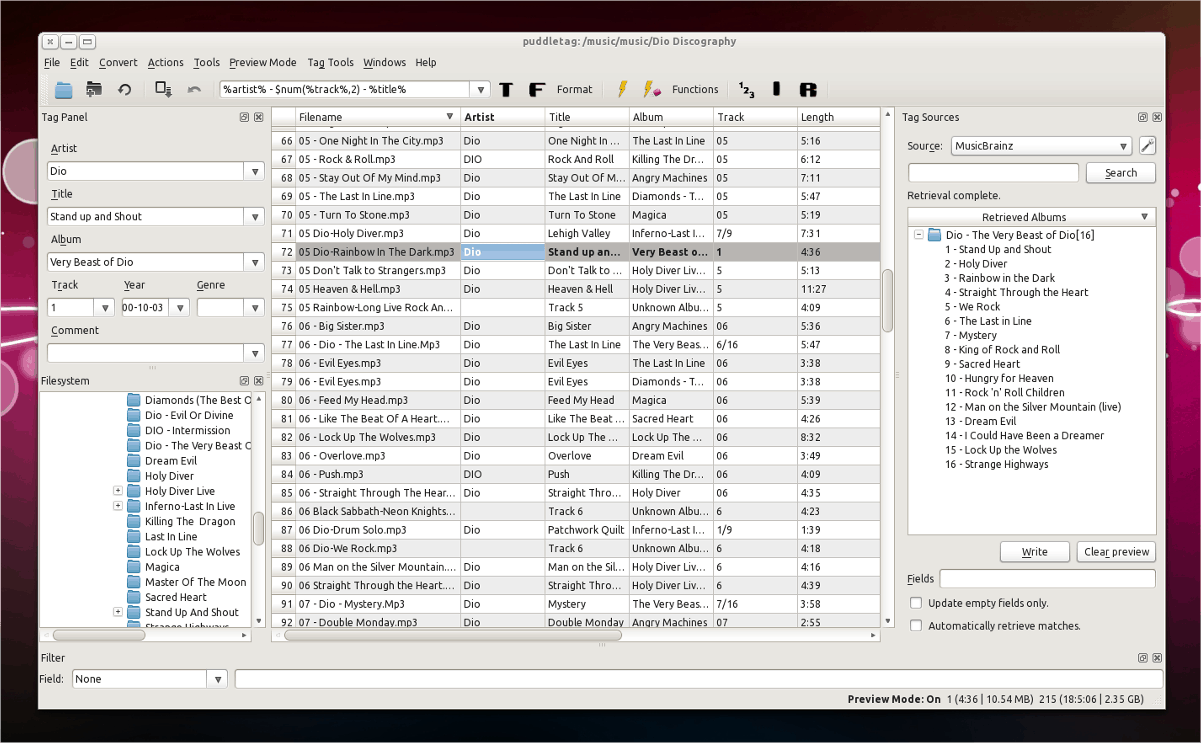
Forward-mode Automatic Differentiation (Beta).(beta) Channels Last Memory Format in PyTorch.(beta) Building a Simple CPU Performance Profiler with FX.(beta) Building a Convolution/Batch Norm fuser in FX.Real Time Inference on Raspberry Pi 4 (30 fps!).(optional) Exporting a Model from PyTorch to ONNX and Running it using ONNX Runtime.Deploying PyTorch in Python via a REST API with Flask.Language Translation with nn.Transformer and torchtext.Text classification with the torchtext library.NLP From Scratch: Translation with a Sequence to Sequence Network and Attention.NLP From Scratch: Generating Names with a Character-Level RNN.NLP From Scratch: Classifying Names with a Character-Level RNN.Language Modeling with nn.Transformer and TorchText.Speech Command Classification with torchaudio.Optimizing Vision Transformer Model for Deployment.Transfer Learning for Computer Vision Tutorial.TorchVision Object Detection Finetuning Tutorial.Visualizing Models, Data, and Training with TensorBoard.
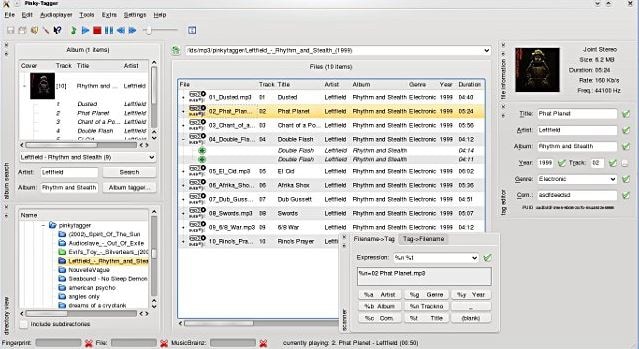
#Finetune automatic music tagger series#
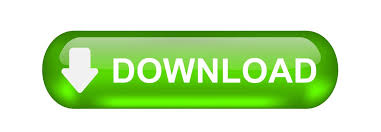